Predictive Analytics
Predictive analytics is a branch of data analytics that involves the use of statistical algorithms, machine literacy ways, and data mining to dissect literal data and make prognostications about unborn events or issues. This type of analysis generally involves working with large datasets and using colorful modeling ways to identify patterns and connections in the data, which can also be used to make prognostications about future trends or actions.
analytics can be used in a wide range of diligence and operations, similar to fiscal soothsaying, healthcare, marketing, fraud discovery, and force chain operation. By using prophetic analytics, associations can gain precious perceptivity into client geste, request trends, and functional effectiveness, which can help them make further informed opinions and ameliorate their business issues.
For illustration, businesses may use prophetic analytics to read unborn deals, identify client parts that are most likely to respond to a marketing crusade, or prognosticate which guests are at threat of churning. In healthcare, prophetic analytics can be used to identify cases that are at high threat of developing certain conditions, allowing for early intervention and better issues. Overall, prophetic analytics is an important tool for businesses and associations looking to make data-driven opinions and gain a competitive edge.
Type of Predictive analytics
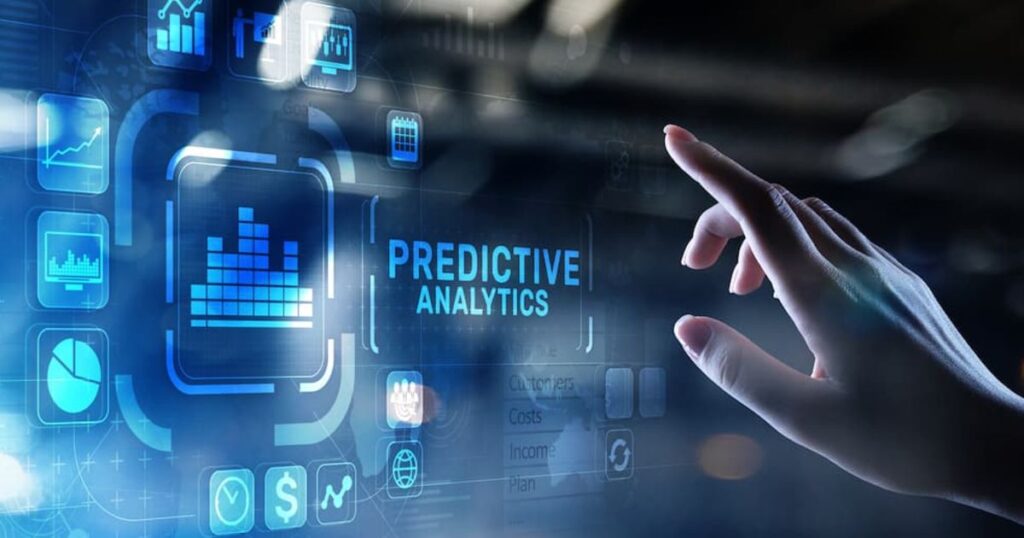
There are several types of predictive analytics, including
Descriptive Analytics: This type of predictive analytics uses data mining techniques to identify patterns and relationships in historical data.
Diagnostic Analytics: This type of predictive analytics focuses on understanding why something happened in the past. It involves analyzing data to identify the root cause of a particular event or outcome.
Predictive Analytics: This is the most common type of Predictive analytics, which involves using statistical models and machine literacy algorithms to make prognostications about unborn events or issues. This can include predicting customer behavior, forecasting sales, and identifying potential risks or opportunities.
Prescriptive Analytics: This type of predictive analytics combines historical data with real-time data to make recommendations or suggestions on what actions to take.
Decision Trees: This technique involves creating a tree-like model of decisions and their possible consequences. It is often used in customer segmentation and risk analysis.
Association Rule Mining: This technique is used to identify patterns and relationships between variables in large datasets. It can be used to identify which products are often purchased together or to find relationships between different medical conditions.
Text Mining: This technique involves analyzing large amounts of unstructured text data, such as customer reviews or social media posts, to identify patterns and insights. It can be used to predict customer sentiment or to identify emerging trends in a particular industry.
Ensemble Methods: This technique involves combining multiple predictive models to improve accuracy and reduce errors. It can be used to create more accurate and robust predictive models.
Anomaly Detection: This technique is used to identify outliers or anomalies in data that do not conform to expected patterns.
Uses of Predictive analytics
Predictive analytics has many practical uses across different industries and sectors. Here are some common use cases:
Marketing: analytics can be used to identify and target potential customers with personalized marketing messages. This can increase the effectiveness of marketing campaigns and improve customer engagement.
Healthcare: analytics can be used to identify patients who are at risk of developing certain conditions or diseases, allowing for early intervention and better outcomes.
Finance: analytics can be used to forecast market trends, identify investment opportunities, and reduce financial risks.
Customer Service: analytics can be used to identify customer needs and preferences, allowing organizations to provide personalized support and improve customer satisfaction. It can also be used to predict customer churn and take proactive measures to retain customers.
Supply Chain Management: Predictive analytics can be used to optimize inventory management, reduce waste, and improve logistics. It can also be used to predict demand and adjust production accordingly.
Human Resources: Predictive analytics can be used to identify high-performing employees and predict employee turnover. It can also be used to improve hiring decisions and develop more effective training programs.
Risk Management: Predictive analytics can be used to identify potential risks and assess their likelihood and impact.
Operations: Predictive analytics can be used to optimize operational efficiency and reduce downtime. It can also be used to predict maintenance needs and prevent equipment failures.
Energy Management: Predictive analytics can be used to optimize energy consumption and reduce costs. It can also be used to predict energy demand and adjust supply accordingly.
Sports Analytics: Predictive analytics can be used in sports to prognosticate game issues, identify implicit injuries, and ameliorate player performance. It can also be used to optimize team rosters and game strategies.
Fraud Detection: Predictive analytics can be used to descry fraudulent conditioning in real-time, similar as credit card fraud or insurance fraud. It can also be used to identify patterns and trends in fraudulent activities to prevent future occurrences.
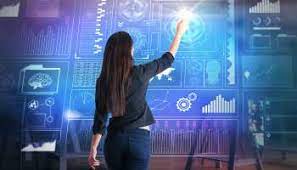
[…] Predictive analytics […]
[…] Read…Predictivhttps://techcordial.com/blog/technology/data-science/predictive-analytics/e […]
[…] Read Also…Predictive Analytics […]
[…] Also Read ….Predictive Analytics […]